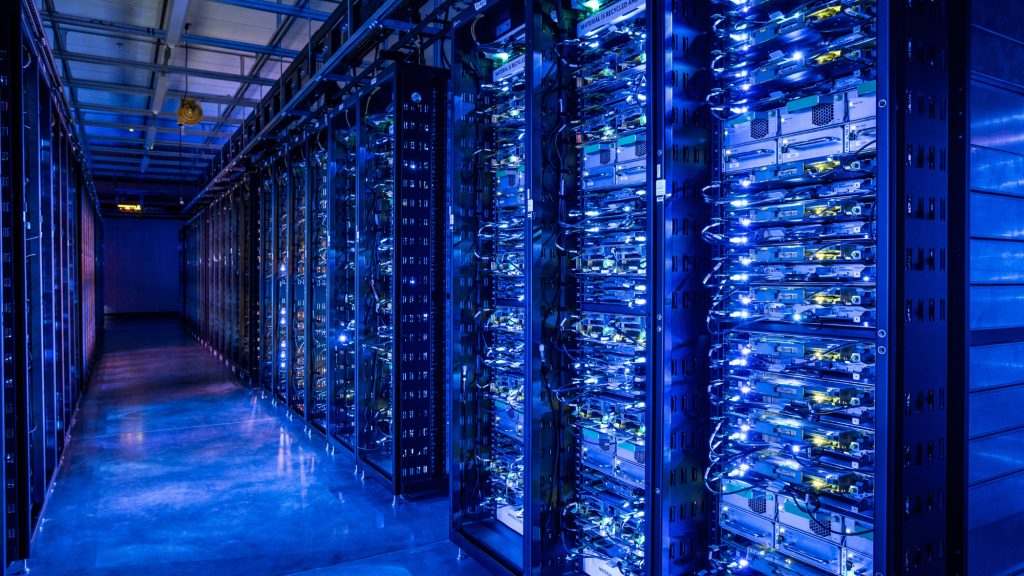
Abstract:
What is Generative AI? The concept of generative artificial intelligence and terms like “AI” have gained an unprecedented level of hype as well as misunderstanding in the past few years; “AI” has become an all-encompassing buzzword to describe complex concepts varying from lengthy analysis of medical data to the generation of memes. But what is it? In a sentence, generative artificial intelligence is the output achieved from complex webs of probability-reliant algorithms called neural networks, designed on the basis of the human brain. When trained with massive amounts of data, these neural networks have the capability to produce human-like responses, in some cases as or more complex and comprehensive as human work. The technology’s hype has skyrocketed due to rapid industry developments and massive investment into generative AI tools like OpenAI’s ChatGPT and Google Gemini. As a result of these popular large language models (LLMs), AI fever has spread throughout nearly every industry in advanced nations like the United States from banking[2] to agriculture[3]. However, the consequence of this accelerated growth in recent years has been society’s blindness to the severe costs of our new favorite toy. An important detail that is increasingly underrepresented in public media is the environmental impact associated with the scaled use of artificial intelligence. More specifically, in the United States, a majority other than the creators of these tools are generally ignorant to resources they consume. Powerful AI models require massive amounts of electricity and water, which contributes to an increasing strain on resources. Further, this massive increase in electricity demand has serious implications for greenhouse gas and CO2 emissions. What is thought to be a fascinating glimpse of a bright future may be a cause for concern that our climate crisis will only continue to spiral out of control. This paper serves to analyze the uses of artificial intelligence today, the energy requirements and environmental impact of AI, and what industry growth will mean for our future energy needs.
Introduction:
I can vividly remember my first interaction with ChatGPT. In 2022 I was sixteen years old; I recall the word spreading like wildfire around my high school of a brand new cheat, a revolutionary way to avoid doing your homework. Like it was magic, a simple prompt into ChatGPT would elicit entire works of writing within seconds. Immediately I could see that our world was about to change. However, after my initial awe of the technology faded, an uneasy feeling remained. Though I could not place it then, I knew that there was something wrong behind the shiny facade of generative AI.
Still, since then it appears that artificial intelligence has been selected as the next champion industry for the United States as well as other developed nations; According to Statista[4] and Axios[8], the U.S. AI market has doubled in valuation since 2020 from $25bn to >$50bn in 2024. Additionally, as of August 2024, there were already over 200 million weekly users of AI tools like ChatGPT, more than doubling since November 2023. Beyond this, AI usage as a whole has jumped 21% since last 2023. For context, not all of the total AI usage is generative AI: the implementation of AI has many different forms from data analysis to language transformation. However, all artificial intelligence tools, in particular GenAI systems like GPT, use complex neural networks that are powered by advanced computers and processors. It is within these processors and data centers that serious environmental costs are found. High-powered GPUs or Graphics Processing Units initially used for videogames and image processing, power many data centers on which AI models are built. These data centers and their GPUs require exorbitant amounts of electricity produced from coal and natural gas to perform computations. According to a report on AI energy consumption from Goldman Sachs[5], just one question to ChatGPT uses 10 times more electricity than a standard Google search, despite achieving very similar outputs. Extending upon this, Google has now incorporated generative AI into every search with the “AI Overview”, meaning that whether you like it or not, your search is using the highest amount of electricity. Further, likely the most important factor is that these GPUs cannot be turned off, meaning that electricity and fresh water for cooling are in twenty-four-seven demand. Despite the billions of dollars being poured into AI, no dollar valuation is enough to cover the cost of increasing CO2 emissions and the use of finite, clean drinking water. There is a public and private sentiment that generative AI is purely beneficial and should be prioritized regardless of potential downsides; This widely held, ignorant optimism is unfortunately not a full nor correct school of thought. While the potential of artificial intelligence down the road is potentially incredible, the unfortunate truth is that operating with our current practices, we may never fully reach it due to environmental limits. Continued development and implementation of AI without drastic improvements in sustainability will lead to the rapid depletion of our natural resources and accelerated destruction of Earth’s environment.
Electricity Consumption of AI:
The most significant detriment to the environment from artificial intelligence comes in the form of the gargantuan electricity requirements associated with training and using AI models. It is not necessarily training that is the most harmful; While training only occurs once, the final model can be used billions of times, often more than once per session by each user.
To get a better understanding of how artificial intelligence is negatively impacting our environment we must quantify these requirements; This task becomes difficult rather quickly due to the recency of AI development and a lack of reporting on AI’s energy consumption. Only the most recent research has data to quantify this environmental impact, for example, a section in the 2024 Neurocomputing scientific journal titled: “A review of green artificial intelligence: Towards a more sustainable future.”[6]. One specific excerpt from this journal that seems to quantify the impact of generative AI reads, “This energy consumption is projected to potentially reach over 30% of the world’s total energy consumption by 2030.3 Large language models (LLMs), such as the recently launched ChatGPT (with GPT-4 as the backbone model) aggravate this trend with their substantial energy requirements. Some authors [1] have estimated that training GPT-3 on a database of 500 billion words required 1287 MWh of electricity and 10,000 computer chips, equivalent to the energy needed to power around 121 homes for a year in the USA. Furthermore, this training produced around 550 tons of carbon dioxide, equivalent to flying 33 times from Australia to the UK. Since the subsequent version, GPT-4, was trained on 570 times more parameters than GPT-3, it undoubtedly required even more energy.”
In addition to this powerful quote, the following graph serves to visualize just how damaging these generative AI models can be as a result of training alone.
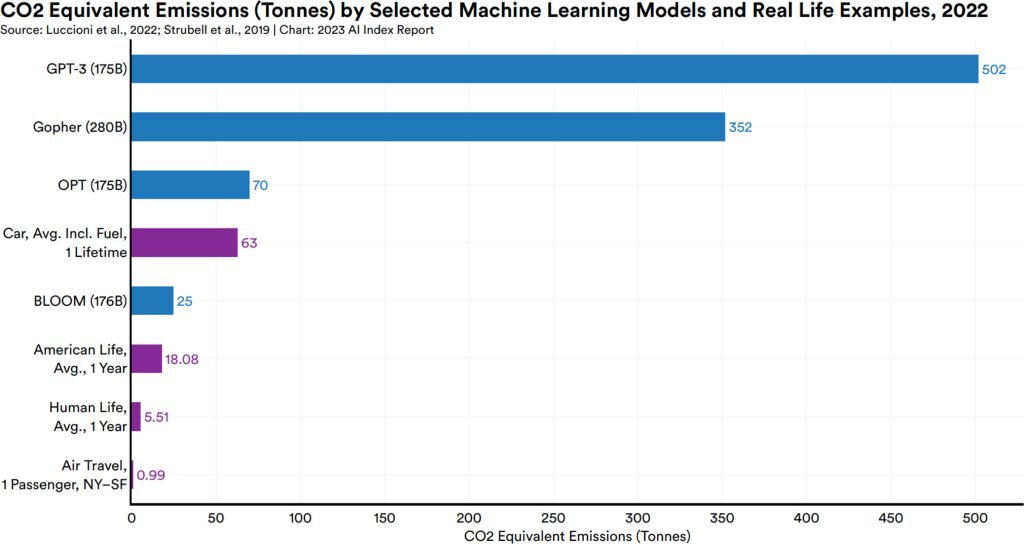
These figures and others like them included in Neurocomputing help bring to light the reality of the rampant energy consumption that occurs with the new technologies included in almost every industry today. Already, GPT-4 and other similar LLMs have had immense impacts on the environment in the form of carbon emissions that contribute to climate change. However, the key detail alluded to in the journal is not the energy usage and emissions that have already been released, but instead, the exponentially greater damage that will be done in the future with the continued growth of the generative AI field.
One statistic in particular that puts the electricity consumption of AI systems into perspective in an accessible way comes from a piece by J.P. Morgan’s Europe Equity Research, in a review entitled, “Avoiding “NucleHype”. An Optimistic Reality Check on the AI Driven Nuclear Revival”[7]. The emboldened text reads, “AI is adding another “Japan” to electricity demand in the next 3 years:” followed by, “In its 2024Electricity Report, the International Energy Agency (IEA) highlights that data centers, cryptocurrencies and AI account for about 2% of global electricity demand, and that rapid data center expansion could double its electricity consumption from 460TWh to more than 1000TWh in 2026, i.e. adding another Japan to global demand in a few years.” This analysis provides a perfect way to emphasize the scale of AI’s electricity demand. As development and implementation continues, AI technology alone is ready to account for a serious share of global electricity consumption. Consequently, due to the inherent connection between current electricity demand and carbon emissions, AI is joining the table as one of the most significant emitters in global industry.
Water Consumption for Cooling:
The energy implications of artificial intelligence are obvious; Electricity requirements to power data centers and run computations are enormous and are causing serious ramifications for the environment in the form of CO2 emissions. However, this is not the only significant harm to the environment associated with artificial intelligence. The use of freshwater for cooling is neck-and-neck with AI’s electricity consumption, fighting for the spot as the most negative side effect of the new technology taking the world by storm. As described in an article published by OECD.AI[8], a global hub for AI policy and regulation, drinking water is used in two different ways during the process of artificial intelligence generation.
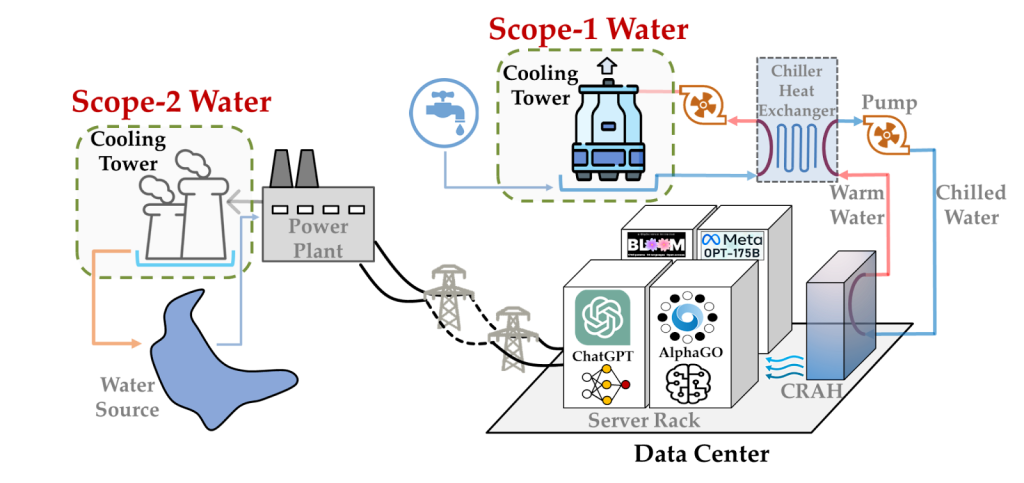
First, freshwater is used in the production of electricity, a pivotal resource for artificial intelligence. Power plants, both thermal and nuclear, require water for evaporative cooling, represented by “Scope-2 Water” in the visual. The second, more integrated use of water comes in the data center itself, where water is used to cool computers and prevent overheating. This process of cooling continues twenty-four hours a day as GPUs and data centers cannot be turned off. Because of this, fresh drinking water is used continuously by AI companies for both electricity and GPU cooling.
How much water can they really be using though? The answer is a lot. According to the same source, OECD.AI, training a large language model (LLM) like GPT-3 can consume millions of liters of fresh water. And yet, training is just the beginning; The real damage is done only once the model is in use. Every 10 questions input into a chatbot like GPT uses around 500mL or a tenth of a gallon(0.132086g) of water. According to an article by Axios[9], OpenAI reported in August 2024 that ChatGPT had over 200 million weekly users, more than double the number from less than a year before. Therefore, conservatively assuming that each user asks a single question per month, that would equate to 2.6 million(0.13208 x 200,000,000) gallons of fresh drinking water per week.
As implied by ChatGPT’s user increase, the use of fresh water for cooling is only increasing. Another example of increased water consumption comes from an article published by the Yale School of Environment[10] in which researchers detail the idea of water usage for AI. In particular, one figure from the article stands out. “Google’s data centers used 20 percent more water in 2022 than in 2021, while Microsoft’s water use rose by 34 percent.” This figure serves to quantify the increase in water consumption by large tech companies in the age of artificial intelligence. However, this figure does not reflect how this consumption has increased in the years since, which has seen more development and investment into AI than ever before. Certainly, the use of freshwater has only increased by more since this article’s release.
Do the Benefits Outweigh the Costs?:
Yet, even considering the resource-intensive nature of this technology, there is a reason that AI has been incorporated into almost every function of daily life for many people on Earth. Many will attest that artificial intelligence has the potential to be the most impactful advancement and most empowering technology in human history. To be fair, there is a degree of truth to this statement. Artificial Intelligence as a technology is not limited to the generative AI tools that are popular in mainstream media; As a whole, it is already one of the most important inventions to date. In the following article about the history of artificial intelligence[11], the broad uses and capabilities described make a case for this technology. Every time we write a text, drive a newly released car, search for something using Google, buy an airline ticket, or apply for a loan, artificial intelligence comes into play during the process. Since the early stages of computers in the 1950s and the first neural network in 1957, artificial intelligence of every kind has elevated our technological capabilities and standard of living as it has progressed. One fascinating example of how analytical machine learning has been able to improve human living and aid the global climate crisis can be found in the Engineering Science & Technology Journal in a section titled “Reviewing the role of artificial intelligence in energy efficiency optimization.”[12] This section of the Nigerian scientific journal delves into the application of machine learning in energy optimization and allows a sneak peek into how artificial intelligence may help us dig our way out of the current climate crisis. A particular passage that exemplifies this reads, “Google’s DeepMind AI was used to optimize the cooling systems of its data centers. By analyzing data from sensors and weather forecasts, the AI system was able to reduce energy consumption for cooling by up to 40%. Siemens developed an AI-based energy management system for buildings, which optimizes heating, ventilation, and air conditioning (HVAC) systems based on occupancy patterns and weather conditions. The system has been deployed in several buildings, resulting in significant energy savings.” This passage and the broader story behind it are part of a key argument for the continuation of AI development: the idea that machine learning and automated data processing can be used to decrease energy and water consumption shines a favorable light on the widespread use of AI. While AI is currently a climate net negative, proponents argue that it will help humans evade the climate crisis in the future. However, this passage and the rest of the journal leave out a key piece of information: While the energy consumption of the data center was optimized as a whole, more resources were required to analyze data and optimize the center, so the difference may be nominal. We see this trend with big tech companies a lot: energy efficiency may increase, yet so does total consumption.
Generative AI: Why is it Special?:
However, this paper is specifically about GenAI, not the data processing systems that have existed for decades. Generative Artificial Intelligence or GenAI systems that use LLMs and photo realistic visual imagination are what dominate headlines and attract massive investment in 2024. Additionally, it is these complex generative AI systems that are the most energy-intensive and have the largest impact on the environment.
So, why might Generative AI be worth the environmental costs? The argument for GenAI relies on the innate human desire to maximize efficiency and automate tasks. Just like technological revolutions of the past such as the Industrial Revolution, many claim that the AI Revolution has the potential to improve human productivity and efficiency by leaps and bounds. Instant generation of photorealistic images and video, gathering and interpreting information from any number of sources in seconds, or running thousands of mathematical computations; GenAI can instantly perform tasks that would otherwise take humans days to achieve. For example, using OpenAI’s “ScholarGPT”, one can begin deep research on any subject within minutes. Just type in, “Find the latest research about AI” and watch the machine gather sourced journal articles and data from across the internet, then summarize all of the inputs into easily understandable writing. With these new tools, if humans ever lack inspiration, GenAI can kickstart productivity and set a higher standard of excellence for human creativity. However, GenAI is not just a tool to spark your creativity when you hit writer’s block. There are real ways to use generative AI to automate laborious tasks and revolutionize productivity. An example of one of the best current uses for GenAI lies in its implications for coding. What was once a tedious and skill-intensive task, only reserved for the smartest computer nerds, can now be nearly entirely automated and is accessible to more people than ever. In an article published by IBM[13], an established company and leading proponent of the AI wave, they detail the uses of AI in code generation. “By infusing artificial intelligence into the developer toolkit, these solutions can produce high-quality code recommendations based on the user’s input. Auto-generated code suggestions can increase developers’ productivity and optimize their workflow by providing straightforward answers, handling routine coding tasks, reducing the need to context switch and conserving mental energy. It can also help identify coding errors and potential security vulnerabilities. —Programmers enter plain text prompts describing what they want the code to do. Generative AI tools suggest code snippets or full functions, streamlining the coding process by handling repetitive tasks and reducing manual coding.” According to IBM, programmers can now generate code as well as have AI fix bugs automatically. While specific to coding, this article exemplifies the phenomenon of AI’s boost to human productivity. No longer will coding be a difficult, mucky path that drags the creative process; Generative AI will allow humans to automate coding and lead to more coding, more projects, and increased innovation.
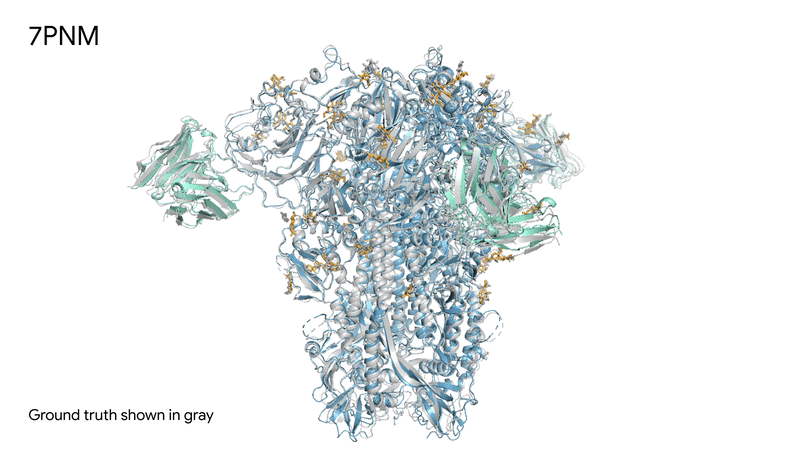
Expanding upon this, it seems that the uses for generative AI in current industries are growing by the second. The most riveting example to date of generative AI being implemented in industry may be the use of deep learning to generate protein structures with Google AlphaFold. In this case, neural networks trained on massive amounts of medical and protein structure data can render 3D models usable for pharmaceutical research and drug development. A 2024 journal titled: “Review of AlphaFold 3: Transformative Advances in Drug Design and Therapeutics”[14] published in the National Library of Medicine discusses the potentially profound capabilities of Google AlphaFold 3. Specifically, one quote that summarizes the excitement around AlphaFold reads, “Its ability to predict the structure of protein-molecule complexes, including those containing DNA and RNA, marks a significant improvement over existing prediction methods. This capability is especially valuable for drug discovery, as it aids in identifying and designing new molecules that could lead to effective treatments.” Essentially, this journal serves to highlight the astounding nature of this technology and its future applications, as well as the significant improvement in this iteration from AlphaFold 2. The reason why scientists and pharmaceutical companies are so excited about this revelation is the potential that it holds for speeding up the research process as well as cutting costs by phasing out current, more expensive methods. Before AlphaFold, researching protein structures was a process that in certain cases took years to complete; Now, it might just take seconds. Additionally, beyond just rendering models, AlphaFold 3 is capable of showing the impacts of chemical modifications on proteins which is crucial for drug development. Now, while the authors acknowledge that this technology is not yet perfectly accurate, the significance of this technology lies in the potential to fully automate certain medical research. If achieved, pharmaceutical industries could see a massive increase in research and production of new treatments. This technology is one of the leading examples of how generative AI is changing human productivity and quality of life.
AI Generation of Photo and Video:
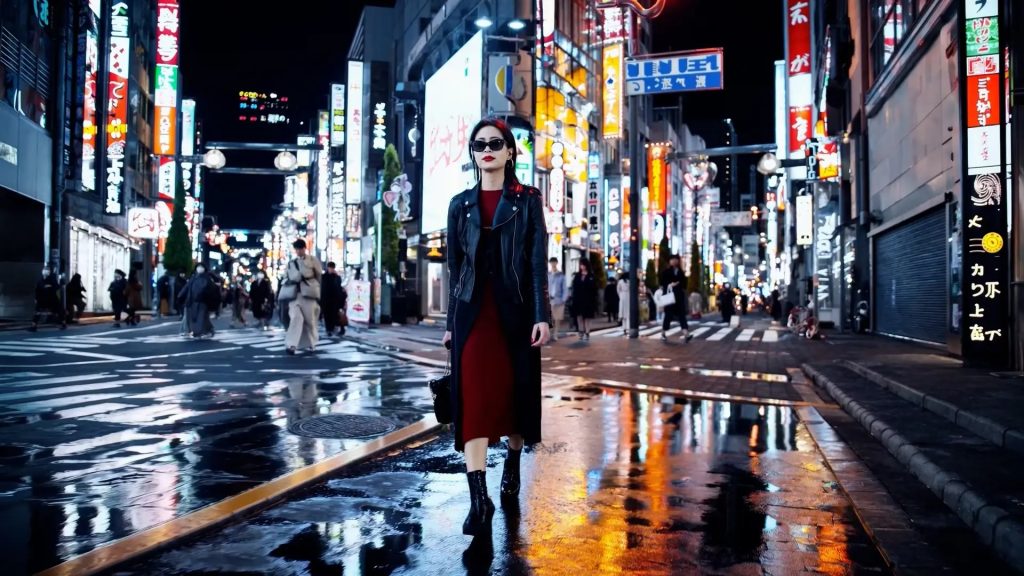
An analysis of generative artificial intelligence would hardly be complete if it failed to include perhaps the most polarizing capability of GenAI: the generation of photorealistic images and videos from learned data. Additionally, this section is separated from my analysis of language and code generation because the visual imagination side of GenAI has almost entirely separate applications. Beyond that, it is this form of GenAI that has by far the most potential for harm, both environmental and social..
In the past year or so, knowingly or unknowingly, everyone who uses the internet has likely come across some type of AI-generated photo or video. These generated images have become unavoidable and just by looking at them it is easy to see the fascination with how eerily similar they are to real photos. Yet, they are not real. The development of this technology has created a space for significant social detriment in the form of disinformation, fake news, and creating a separation from reality. One argument often used in favor of photo and video generation is that this technology will be a tool to increase productivity for artists, graphic designers, and media production. Like with other forms of GenAI, it is true that productivity indeed stands to gain. However, with the generation of photos and videos, there is less tangible work being produced and much more at risk. Generating nifty photos for entertainment is not worth the potential collapse of information.
Beyond that, the energy and water implications of photo and video generation are monstrous, even against language generation. Due to the sheer complexity of the neural networks used to create these visuals that outpace any other form of GenAI, the environmental impacts are far greater than text generation. In an article by the MIT Technology Review[15], a glimpse into the environmental impact of visual generation is possible. “Generating an image using a powerful AI model takes as much energy as fully charging your smartphone”, the article reads, highlighting just how energy-intensive this process can be. More importantly, with electricity usage, there are automatically carbon emissions related. This article acknowledges this fact as it writes, “Generating images was by far the most energy- and carbon-intensive AI-based task. Generating 1,000 images with a powerful AI model, such as Stable Diffusion XL, is responsible for roughly as much carbon dioxide as driving the equivalent of 4.1 miles in an average gasoline-powered car.”
As presented by this MIT study, the generation of visuals using advanced neural networks is by far the most energy-intensive form of AI and thus the most harmful to the environment when operating at scale. Moreover, all of this detriment to the environment is arguably unnecessary, as visual generation does not add nearly as much to human productivity as language generation. The environmental and social concerns of this form of GenAI make it quite difficult to advocate in favor of.
Is Artificial Intelligence Limited by Energy Boundaries?:
The broad range of uses that I have discussed reflects the massive potential for benefit with GenAI. However, the question remains, does any of it matter? Before indulging in fantastical possibilities of this technology down the line, we must consider whether this potential will ever be realized. The fact remains that the development and growth of AI are reliant on the supply of electricity and water, meaning that AI growth may be limited by environmental boundaries. According to the Energy Information Administration (EIA)[17], 60% of electricity in the United States is still produced from fossil fuels, with 40% of electricity being generated from natural gas. According to previously sourced research, AI energy usage could potentially account for 30% of total electricity demand by 2030, which to an estimate from Statista[18] could be from 31,000-36,000 TWh of electricity, meaning that AI energy demand could reach anywhere from 9,000-11,000 TWh of electricity by 2030. However, there is only so much electricity that can be produced by our current methods. There is only so much coal and natural gas to use for energy production, a little over 50 years for global natural gas according to a study by Swiss energy analytics company METgroup[19]. Additionally, this number is likely inaccurate, as the rate of increase in energy demand is increasing rapidly and is unknown for the future. Nevertheless, using all of our coal and natural gas is not an option. In a 2019 UN Press release, the committee stated that there were 11 years left before irreversible climate change sets in due to the use of fossil fuels. Therefore, by 2030, if we do not change our sources of energy, the damage to our Earth will be irrevocable. This problem is only exacerbated by the exponential growth of artificial intelligence. By analyzing the data presented, one conclusion is impossible to ignore. Despite the amazing potential of advanced computing and GenAI, there is no way to ever see this technology in full form without rapid improvements in energy solutions.
Furthermore, even ignoring these environmental limits, increasing electricity demand will inevitably hit a ceiling that is the capability of the U.S. power grid. According to an article by the National Renewable Energy Laboratory (NREL)[20], “To electrify everything from vehicles to heating systems to stovetops, the U.S. grid must expand by about 57% and get more flexible, too. Solar and wind energy are the renewables most likely to dominate a future clean energy grid. But they are found primarily in remote areas, far from the hubs that need their power. And that is a problem. Today’s transmission system simply is not designed to ingest all that remote power.” Simply put, even if the United States were to magically transition entirely to renewable energy from fossil fuels, the current grid could not handle this electricity nor transport it the distance required. These grid limitations create yet another barrier between us and the potential of AI, as increasing electricity demands cannot be met with the current infrastructure.
Hope for the Future:
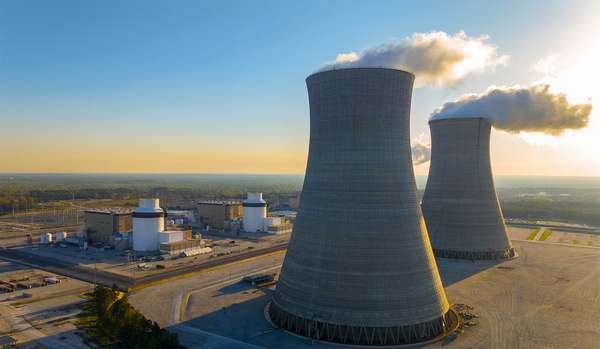
Does the resource-intensive nature of AI and the aging state of the U.S. power grid mean that AI growth is doomed to fail? Fortunately enough, there are solutions that can aid in the protection of the environment and AI’s potential. While the situation is certainly hard to maneuver, there are always opportunities for innovation and examples of companies that are currently operating sustainably. Given the proper planning and the right investment, there is room for the development of AI to continue. One option that has become a popular talking point of improving sustainability in artificial intelligence is the recent trend of nuclear energy in AI. For reference, the 2024 purchase of a Pennsylvania nuclear plant by Amazon Web Services to power a new data center. According to the American Nuclear Society[20], Amazon Web Services, a subsidiary of Amazon and one of the largest data processors as well as users of AI, recently purchased a nuclear power plant to provide clean electricity to a new data center. Several years ago, Amazon issued a goal to reach carbon neutrality by 2040 and hopes to power all operations with renewables by 2025. This purchase is just a part of the overarching environmental goal. Amazon is not the only company getting involved with the nuclear energy field to serve AI energy needs. Microsoft as well as Oklo, a startup backed by OpenAI founder Sam Altman, are other companies moving towards nuclear power for AI growth.
However, while nuclear energy seems like a great solution to the insatiable energy needs of AI, it may not be a viable solution when considering the urgency of this issue. Due to the infrastructure barriers associated with nuclear energy, we must turn to other renewables. While researching this topic, I wanted to understand the industry’s point of view and hear from an expert in AI and renewables. To gain this perspective, I reached out to Dipul Patel, an MIT professor and Chief Technology Officer at Soluna Computing[22]. In our conversation, we discussed the resource usage of AI as well as potential solutions; One key point made by Patel is that the electricity and water demand of AI systems and data centers is directly correlated to how “smart” they are. Logically, the more capable a model or data center is, the more data processing and computing it requires, meaning increased resource usage. As industries invest billions into improving the capabilities of AI, the faster our energy and climate crisis accelerates. So, what is the solution? My next question for Patel was whether or not nuclear energy is the fix for AI’s increasing energy demand and if AI will be a catalyst for the U.S. to transition to nuclear. His response: “Not even close. You cannot fast-track nuclear.”, Patel states, “Nuclear energy requires infrastructure and complex supply chains, the reason nobody is building nuclear plants is because nobody will buy them.” The takeaway is that there are serious barriers in the form of missing infrastructure and outdated nuclear fission technology that block a large-scale transition to nuclear power, especially in the time frame required. Patel then stated that, instead of nuclear, a better alternative that can be accessed immediately is found with wind and solar energy. In particular, wind energy is the most developed and most scalable form of renewable energy.
Patel’s company, Soluna Computing, is a leading innovator in clean computing with wind energy. Soluna is a Bitcoin mining and artificial intelligence operation that achieves sustainable computing by connecting data centers directly to wind farms. By doing this, Soluna can take advantage of excess electricity that cannot be handled by grids and otherwise goes to waste. As a result, Soluna saves wasted electricity while simultaneously powering energy-intensive data centers with renewable energy. While only one company, Soluna’s business model represents a strong case for a solution to the challenges with AI sustainability and resource supply.
Conclusion:
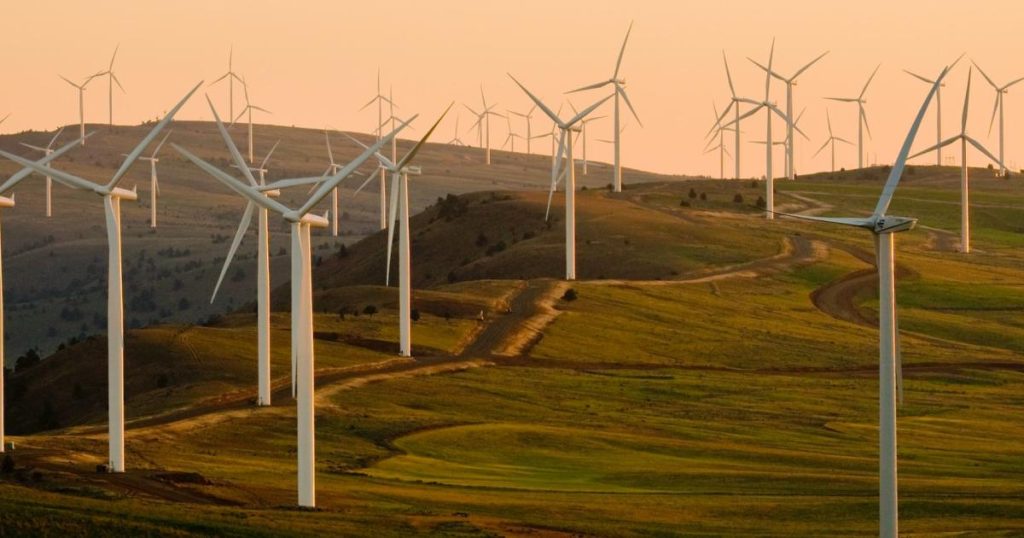
Considering the evidence, it would seem that a grave conclusion exists that artificial intelligence is killing our environment, its growth is doomed for failure, and the end of the world is near. And yet, there are nuances to every conclusion and alternative perspectives to every piece of information.
The purpose of this paper is not to condemn generative artificial intelligence. My goal with this research is not to say that society should halt AI development or abandon the technology. On the contrary, I believe that artificial intelligence is the next step for societal and economic development. Instead, the purpose of this paper is to highlight a significant flaw that is mostly underrepresented and misunderstood. Unless addressed, this flaw will lead to worsened effects of climate change and the eventual death of a revolutionary invention.
The reality is that artificial intelligence, specifically generative AI, is extremely resource-intensive in its use of electricity and fresh water. Due to the excessive electricity demand, AI models are directly correlated with increased carbon emissions from the burning of fossil fuels and therefore global warming. Additionally, the use of water for both the production of electricity and the maintenance of GPUs is a serious threat to the decreasing levels of usable freshwater left on Earth for drinking and agriculture.
Nevertheless, it would be incorrect to state that generative artificial intelligence is purely negative. On the contrary, it holds the potential to be the technology that will accelerate human efficiency and productivity forever. The current applications of AI will only be outclassed by coming iterations, incomprehensible to us today. Additionally, there are already instances in which artificial intelligence operations are being carried out sustainably and where AI is being used to improve the climate crisis.
However, whether we want to admit it or not, this technology is becoming a serious threat to the planet. Without rapid improvement in the energy efficiency and sustainability of AI systems, the sources of electricity for data centers and AI models, and the infrastructure needed to carry out these energy improvements, there is by definition a limit to this technology’s potential. We as a society must confront the truth about AI and take action before it is too late. Wind energy, along with other renewables like solar farming, are the best short-term solutions for clean computing at scale. To avoid accelerating the climate crisis further, it is of the utmost importance to invest in wide-scale wind energy as well as the grid infrastructure required to maximize outputs. If not immediately addressed, the energy consumption of AI will reach overwhelming levels. At that point, either the AI industry or the Earth will succumb to the pressure. Regardless of GenAI’s great potential, the resources required are anything but sustainable. The time is now to make the transition to renewables and upgrade energy infrastructure before it is too late.
References:
- Google Data Center, 2018, https://www.techradar.com/news/explosion-at-a-google-data-center-causes-major-outage
- Forbes, April 2024, “How AI Is Growing Fast On Wall Street”
- Assembly Magazine, June 2023, “John Deere Revolutionizes Agriculture with AI and Automation”
- Statista, Updated March 2024, “Market Insights: Artificial Intelligence”
- Goldman Sachs, May 2024, “AI is poised to drive 160% increase in data center power demand”, https://www.goldmansachs.com/insights/articles/AI-poised-to-drive-160-increase-in-power-demand
- Bolón-Canedo, V., Morán-Fernández, L., Cancela, B., & Alonso-Betanzos, A. (2024). A review of green artificial intelligence: Towards a more sustainable future. Neurocomputing, 128096. https://www.sciencedirect.com/science/article/pii/S0925231224008671
- J.P Morgan, Europe Equity Research, 2024, “Avoiding “NucleHype”. An Optimistic Reality Check on the AI Driven Nuclear Revival”
- OECD.AI, 2023, “How much water does AI consume? The public deserves to know.” https://oecd.ai/en/wonk/how-much-water-does-ai-consume
- Axios, August 2024, “OpenAI says ChatGPT usage has doubled since last year” https://www.axios.com/2024/08/29/openai-chatgpt-200-million-weekly-active-users#
- David Berreby • February 6, et al. “As Use of A.I. Soars, so Does the Energy and Water It Requires.” Yale E360, e360.yale.edu/features/artificial-intelligence-climate-energy-emissions.
- Max Roser (2022). “The brief history of artificial intelligence: the world has changed fast — what might be next?” Published in Our World in Data. https://ourworldindata.org/brief-history-of-ai#article-citation
- Olatunde, T. M., Okwandu, A. C., Akande, D. O., & Sikhakhane, Z. Q. (2024). Reviewing the role of artificial intelligence in energy efficiency optimization. Engineering Science & Technology Journal, 5(4), 1243-1256. https://fepbl.com/index.php/estj/article/view/1015
- IBM, 2024, “What is AI Code Generation?” https://www.ibm.com/think/topics/ai-code-generation#:~:text=Generative%20AI%20can%20also%20translate,by%20transforming%20COBOL%20to%20Java.
- National Library of Medicine, 2024, “Review of AlphaFold 3: Transformative Advances in Drug Design and Therapeutics”, https://pmc.ncbi.nlm.nih.gov/articles/PMC11292590/
- MIT Technology Review, 2024, https://www.technologyreview.com/2023/12/01/1084189/making-an-image-with-generative-ai-uses-as-much-energy-as-charging-your-phone/
- Ooi, K. B., Tan, G. W. H., Al-Emran, M., Al-Sharafi, M. A., Capatina, A., Chakraborty, A., … Wong, L. W. (2023). The Potential of Generative Artificial Intelligence Across Disciplines: Perspectives and Future Directions. Journal of Computer Information Systems, 1–32. https://doi.org/10.1080/08874417.2023.2261010
- https://www.eia.gov/tools/faqs/faq.php?id=427&t=3
- Statista, 2024, Global power consumption forecast by scenario 2050, https://www.statista.com/statistics/1426308/electricity-consumption-worldwide-forecast-by-scenario/#:~:text=According%20to%20a%20recent%20forecast,achieved%20(Achieved%20Commitments%20scenario).
- METgroup, 2024, “When Will Fossil Fuels Run Out?” https://group.met.com/en/mind-the-fyouture/mindthefyouture/when-will-fossil-fuels-run-out#:~:text=Conclusion%3A%20how%20long%20will%20fossil,our%20reserves%20can%20speed%20up.
- NREL, 2024, “The grid can handle more renewable energy, but it needs some help.” https://www.nrel.gov/news/program/2024/the-grid-can-handle-more-renewable-energy-but-it-needs-some-help.html
- American Nuclear Society, 2024, “Amazon buys nuclear-powered data center from Talen”, https://www.ans.org/news/article-5842/amazon-buys-nuclearpowered-data-center-from-talen/
- Soluna Computing, 2024, https://www.solunacomputing.com/
- OpenAI, openai.com, Sora Index, https://openai.com/index/sora/
- Time Magazine, 2024, “Best Inventions of 2024”, https://time.com/7094933/google-deepmind-alphafold-3/
- Politico, 2024, Nuclear Power Plant Photo, https://www.eenews.net/articles/white-house-makes-push-for-large-nuclear-reactors/
- Project Drawdown, 2024, Wind Farm Photo, https://drawdown.org/solutions/onshore-wind-turbines